
Data Analytics
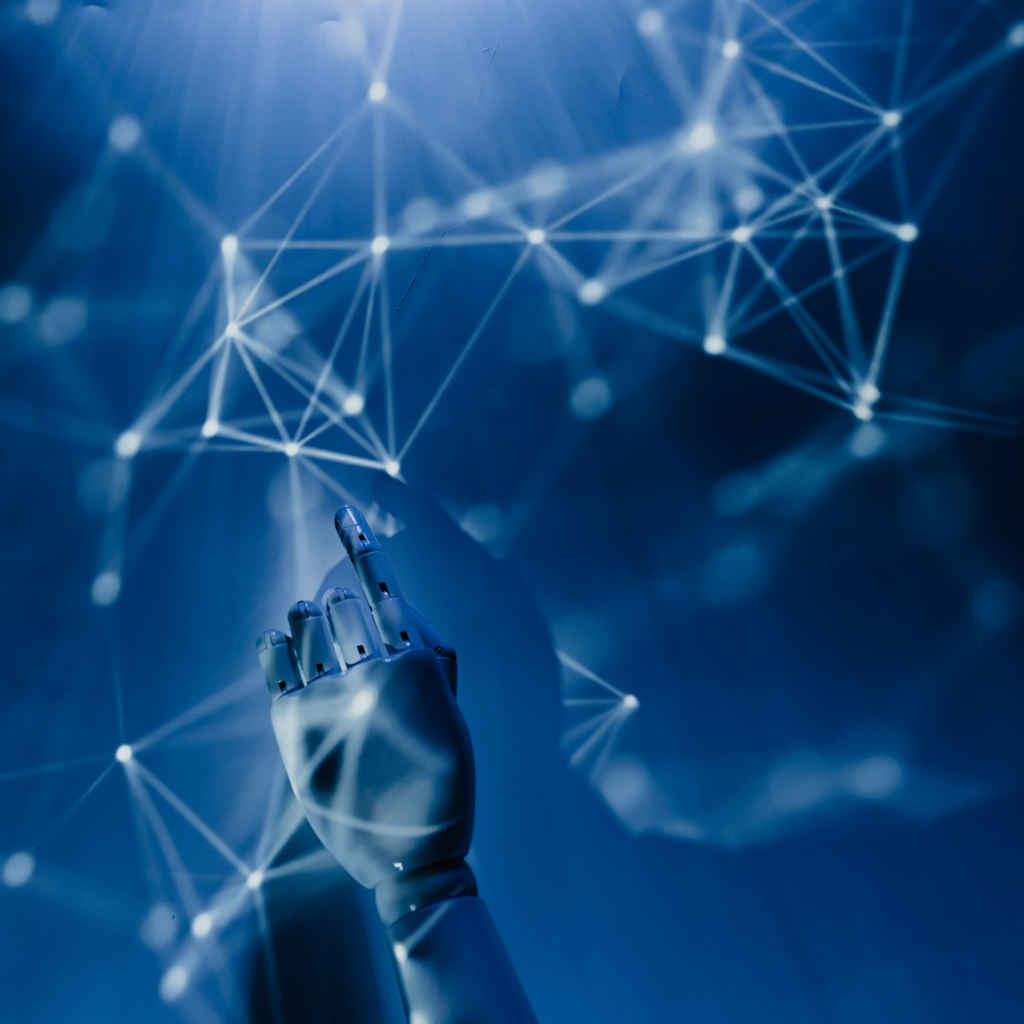
Natural Language Processing
Using NLP techniques, we provide an automated way to create a knowledge graph from the texts from technician service documents. The aim is to create a model that represents the digital twin of a vehicle and to create a feedback loop to update the knowledge graph based on the actions taken by the human (technicians). Why do we need a knowledge graph with feedback loop?
With a footprint of close to 80-90 ECU’s in today’s automotive, and a multitude of trouble codes (often ambiguous), questions are being raised around the ability and effectiveness of traditional Diagnostic tools to accurately diagnose and detect the underlying cause of a given problem/defect.
Improves technician productivity by 60%
The pain-points and the business ramifications are obvious – High warranty costs based on No Fault Found (NFF) instances, Lower Fixed-First-Visit (FFV) rates, Lower technician productivity, High vehicle downtimes, and a cumulative negative bearing on customer satisfaction, retention, and loyalty – With ‘misdiagnosis’ or the ‘lack of diagnosis’ clearly being the primary culprit.
Addresses NFF & FFV challenges
A viable solution in sight is to reduce the reliance on a technician’s decisions and to introduce the concept of intelligent, guided and pin-pointed diagnostics.
Product agnostic
Our Intelligent and graph-based diagnostics solution aims to diagnose with pin-point accuracy, and enable guided & optimum troubleshooting based on Artificial Intelligence (supervised learning), which sits at the heart of this solution.
50% faster problem diagnosis
As add-ons, the sustained buzz and drive for electrification and autonomy of vehicles, coupled with modern engineering approaches such as feature-based architectures, virtual ECUs, etc. are lending an extra layer of complexity to the already complex diagnostics mix.
Predictive Analytics
Given data streamed from a source, like a vehicle—such as diagnostic trouble codes (DTCs) and other vehicle parameters at the time of occurrence of the trouble codes (e.g., odometer reading, vehicle speed, engine temperature, torque, etc.), can we predict an ensuing repair or a maintenance job on the vehicle? We, the Mulytic Labs, developed an innovative solution that is based on On-Board Diagnostic Data from the vehicle and created a diagnostic model, using which our solution can predict what and when different classes of failure might occur in the vehicle. We are answering the business cases to achieve via our predictive analytics solution:
1. Avoiding critical component failure during operations
2. Monitoring the life of the component for re-engineering based on field failures
3. Pre-ordering components to ensure availability at the workshop
Smart Grid Analytics
Enable operating costs, improve grid reliability and deliver personalized energy services to consumers.
Battery Storage Analytics
Ensuring reliability from renewable generation and creating a more flexible transmission and distribution system.
Solar powered system modeling
Ensuring no direct greenhouse gas emissions because the electricity is made from sunlight rather than burning fossil fuels.
Predictive maintenance
Determining the condition of in-service equipment with promising cost savings over routine.
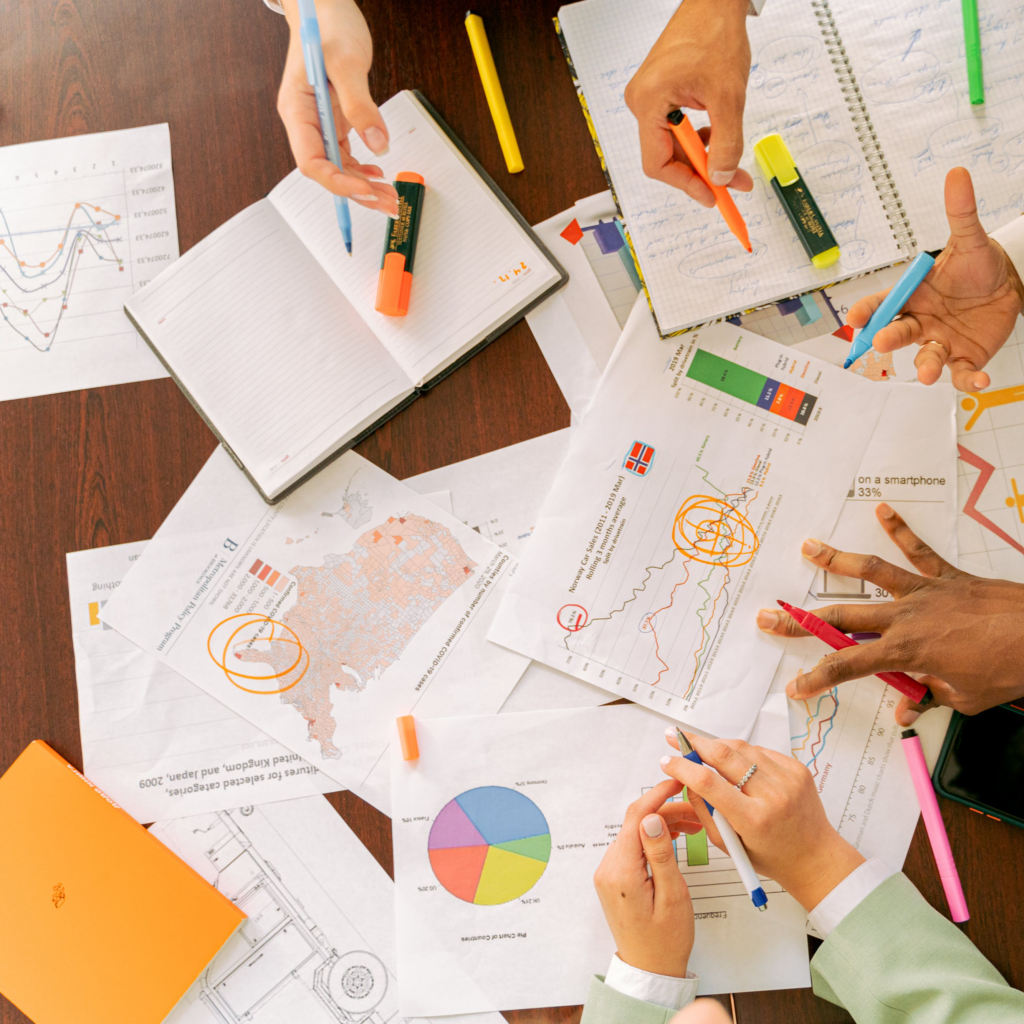

Central Data Lake
A data lake is a central location in which to store all your data, regardless of its source or format. It is typically, although not always, built using Hadoop. The data can be structured or unstructured. You can then use a variety of storage and processing tools—typical tools in the extended Hadoop ecosystem—to extract value quickly and inform key organizational decisions.
Because of the growing variety and volume of data, data lakes are an emerging and powerful architectural approach, especially as enterprises turn to mobile, cloud-based applications, and the Internet of Things (IoT) as right-time delivery mediums for big data.
In broad terms, data lakes are marketed as enterprise-wide data management platforms for analyzing disparate sources of data in its native format. The idea is simple: instead of placing data in a purpose-built data store, we move it into a data lake in its original format. This eliminates the upfront costs of data ingestion, like transformation. Once data is placed into the lake, it’s available for analysis by everyone in the organization.
Unite diverse data sources
A data lake is a centralized repository that allows you to store all your structured and unstructured data at any scale.
Enable self-service
Enabling self-service access to data stored in data lakes has become increasingly complex. We deliver such a sophisticated infrastructure to data users.
Achieve the highest data quality
Everything from collecting the data to making it fit the company’s needs we achieve the highest quality by making it less expensive and time-consuming.
Safeguard through governance
Safeguard enables governments to effectively and efficiently manage the various safety facets of their day-to-day operations.